Generative AI is at a turning point. It’s been over two years since ChatGPT launched, and while the initial excitement about its potential was high, people are now more aware of its limitations and costs.
The AI landscape in 2025 shows this shift. There’s still a lot of buzz—especially around newer areas like agentic AI and multimodal models—but it’s clear that this year will come with some growing pains.
Companies are now looking for proven results from generative AI, not just early-stage prototypes. That’s a tough challenge for a technology that’s often costly, prone to mistakes, and vulnerable to misuse. Meanwhile, regulators will have to find the right balance between fostering innovation and ensuring safety in a rapidly changing tech world.
Here are eight key AI trends to watch out for in 2025.
1. Hype gives way to more pragmatic approaches
Since 2022, generative AI has seen huge interest and innovation, but actual adoption has been slow and uneven. Many companies find it hard to take generative AI projects—whether for internal tools or customer-facing applications—from the testing phase to full use.
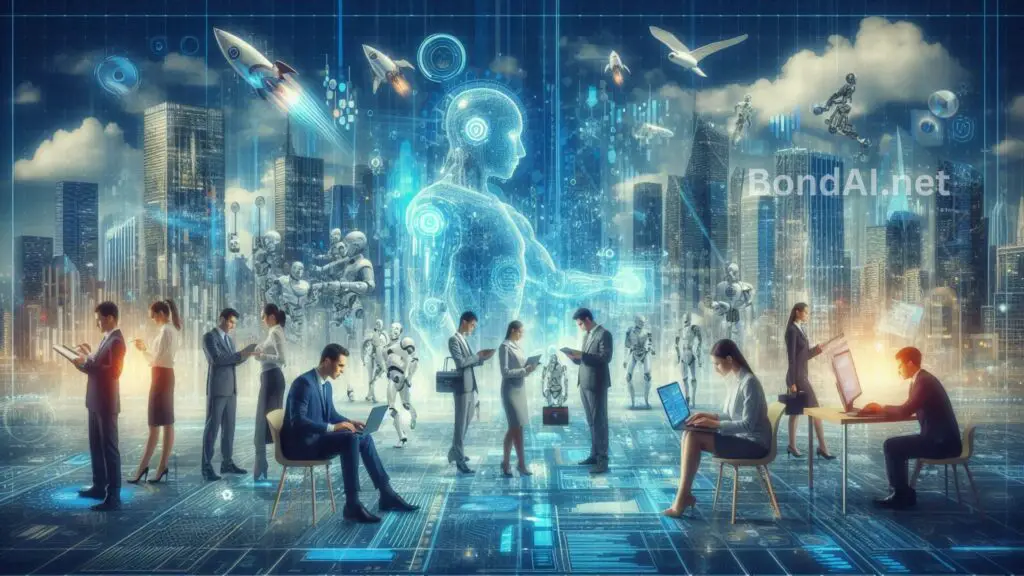
While a lot of businesses have experimented with generative AI through proofs of concept, fewer have fully integrated it into their operations. A September 2024 report by Informa TechTarget’s Enterprise Strategy Group found that, even though over 90% of organizations had increased their use of generative AI in the past year, only 8% considered their efforts fully mature.
Jen Stave, the launch director for the Digital Data Design Institute at Harvard University, was surprised by the lack of adoption. “When you look across businesses, companies are investing in AI, building custom tools, and buying enterprise versions of large language models,” she said. “But we haven’t seen a big wave of adoption within companies.”
One reason for this is AI’s uneven impact across different roles. Organizations are realizing what Stave calls the “jagged technological frontier,” where AI boosts productivity for some tasks but reduces it for others. For example, a junior analyst might become much more efficient using an AI tool, while a more experienced employee might find it a hindrance.
“Managers don’t know where that line is, and employees don’t know either,” Stave said. “So, there’s a lot of uncertainty and experimentation.”
Given the slow pace of adoption, the reality isn’t surprising to anyone familiar with enterprise tech. In 2025, expect businesses to push harder for real results from generative AI: lower costs, measurable ROI, and clearer efficiency improvements.
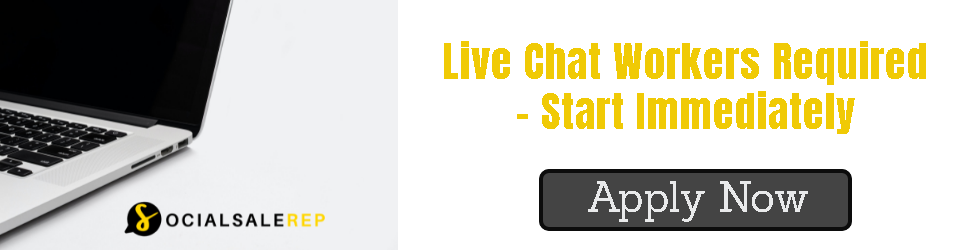
Also Read: How does Jasper AI Compare to Writesonic?
2. Generative AI moves beyond chatbots
When most people hear “generative AI,” they probably think of tools like ChatGPT and Claude, which are powered by large language models (LLMs). Businesses, too, have mainly focused on using LLMs in products and services through chat interfaces. But as the technology improves, AI developers, end users, and companies are starting to look beyond just chatbots.
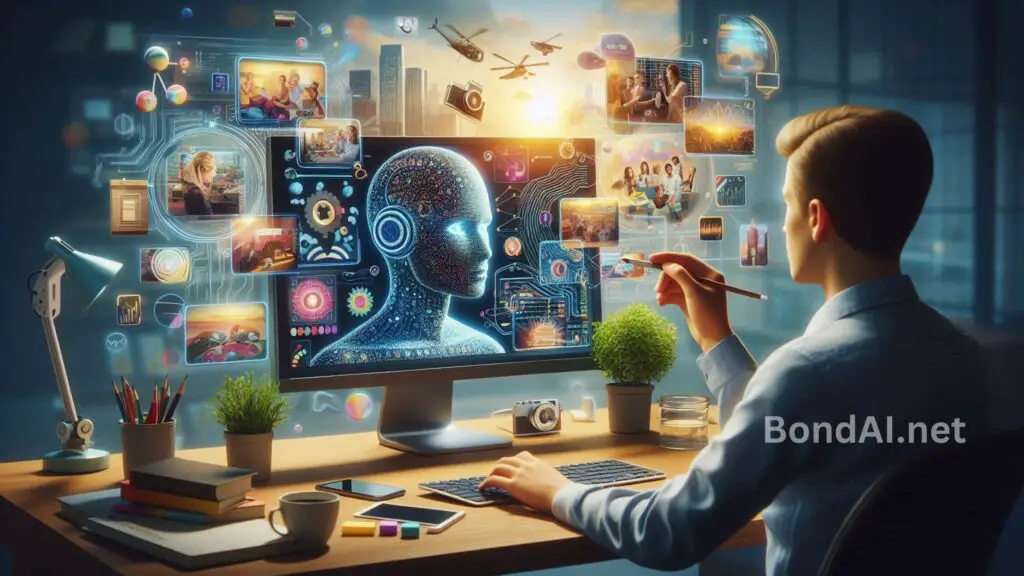
“People need to get more creative with these tools and not just try to slap a chat window on everything,” said Eric Sydell, founder and CEO of Vero AI, an AI and analytics platform.
This shift is part of a larger trend: building software on top of LLMs instead of just using chatbots as standalone tools. Moving from simple chatbots to applications that use LLMs in the background to analyze or summarize unstructured data could help solve some of the challenges of scaling generative AI.
“[A chatbot] can make an individual more efficient… but it’s very one-on-one,” Sydell said. “So, how do you scale that for a whole company?”
Looking ahead to 2025, some AI developments are moving away from text-based interfaces altogether. The future of AI is increasingly focused on multimodal models, like OpenAI’s text-to-video Sora and ElevenLabs’ AI voice generator, which can work with different types of data, like audio, video, and images.
“AI has become synonymous with large language models, but that’s just one type of AI,” Stave said. “The multimodal approach is where we’ll see some big breakthroughs.”
Robotics is another area where AI is moving beyond text. With robotics, AI interacts with the physical world, and Stave believes that foundation models for robotics could be even more revolutionary than generative AI itself.
“Think about all the ways we interact with the physical world,” she said. “The possibilities are endless.”
3. AI agents are the next frontier
In the second half of 2024, there’s been a growing interest in agentic AI models that can take independent action. Tools like Salesforce’s Agentforce are designed to handle tasks for business users, such as managing workflows, scheduling, and data analysis—all on their own.
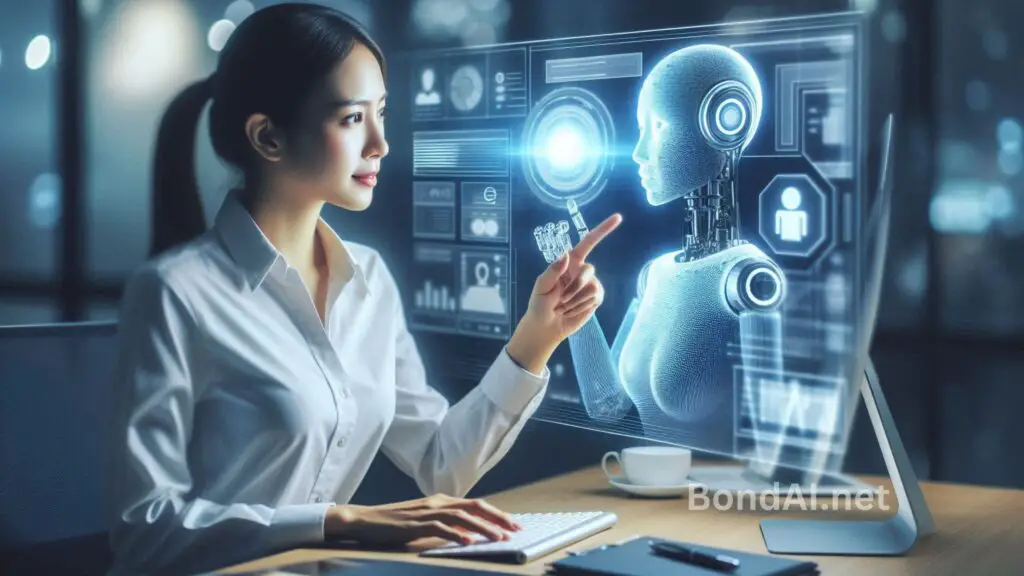
Agentic AI is still in its early stages. While human guidance and oversight are still important, these AI agents can only perform a limited range of actions. But even with these restrictions, they’re appealing across many industries.
Autonomous functionality isn’t exactly new. It’s been a key part of enterprise software for a while. But the real difference with AI agents is their ability to adapt. Unlike basic automation tools, agents can adjust to new information, handle unexpected challenges, and even make their own decisions.
However, this independence comes with new risks. Grace Yee, senior director of ethical innovation at Adobe, pointed out the potential harm that could occur if agents start making decisions on our behalf—like scheduling or completing tasks.
Since generative AI tools can be prone to “hallucinations” (generating incorrect information), there’s a concern about what could happen if an autonomous agent makes similar mistakes with real-world consequences.
Sydell echoed these concerns, mentioning that some uses of AI will raise more ethical issues than others. “When you get into high-risk areas—things that could hurt or help people—the standards need to be much higher,” he said.
4. Generative AI models become commodities
The world of generative AI is changing fast, with foundation models becoming pretty common. As 2025 begins, the focus is shifting from which company has the best model to which businesses are great at fine-tuning existing models or building specialized tools that work on top of them.
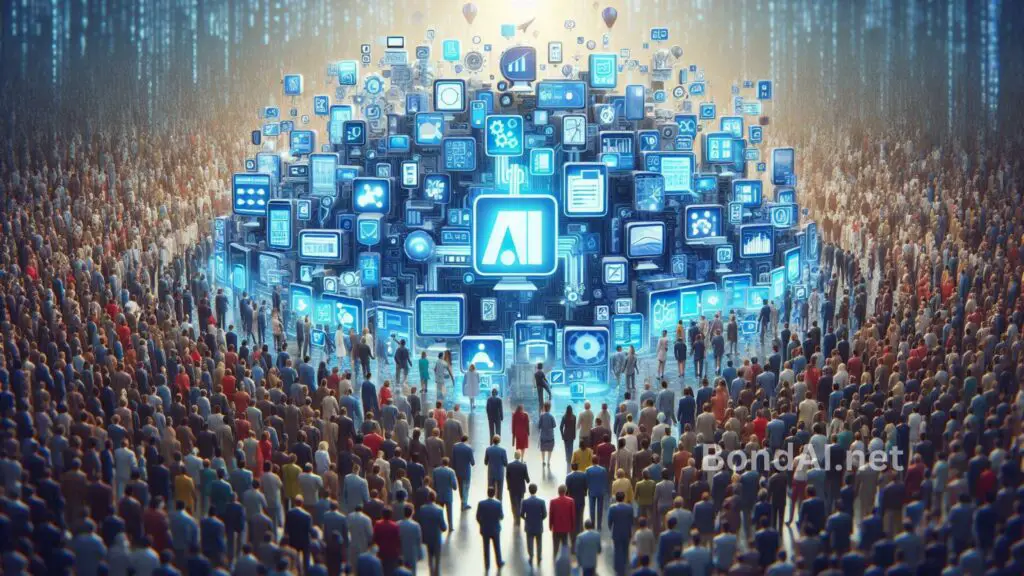
In a recent newsletter, analyst Benedict Evans compared the rise of generative AI models to the PC industry in the late 1980s and 1990s. Back then, people compared computers based on small improvements like CPU speed or memory, just like how today’s AI models are often judged on specific technical benchmarks.
But over time, those small differences faded as the market reached a certain standard. What mattered more was things like cost, user experience, and how easily the product could be integrated. It looks like foundation models are heading in the same direction: As performance levels out, more advanced models are starting to feel interchangeable for many uses.
In a world where models are becoming a commodity, the focus isn’t just on the number of parameters or a slight performance edge. Instead, businesses are prioritizing usability, trust, and how well the AI works with existing systems.
In this environment, AI companies with strong ecosystems, easy-to-use tools, and competitive prices are the ones most likely to come out on top.
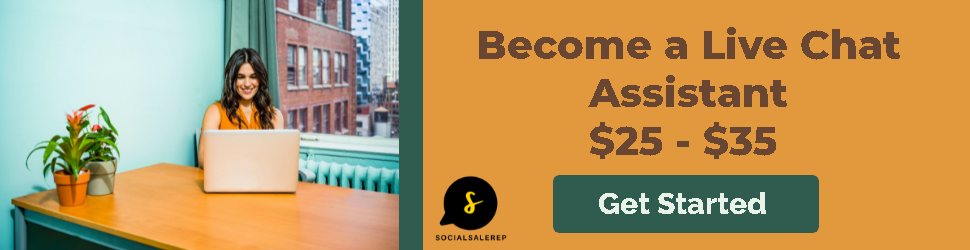
Also Read: How is AI Shaping the Future of Education?
5. AI applications and data sets become more domain-specific
Top AI labs like OpenAI and Anthropic are working toward the big goal of creating artificial general intelligence (AGI), which is basically AI that can do anything a human can. But for most businesses, AGI — or even today’s foundation models — isn’t really necessary.
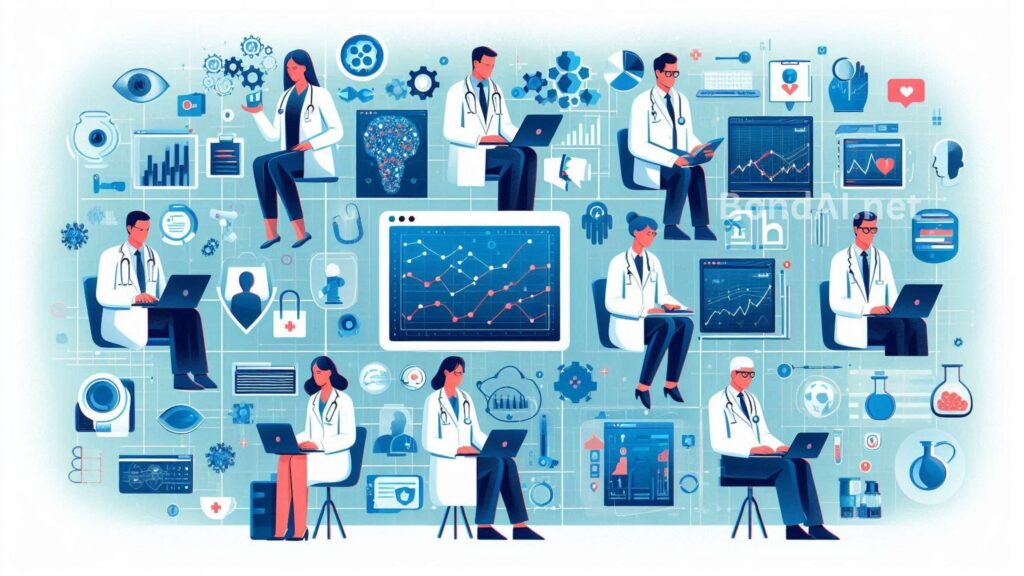
From the start of the generative AI boom, businesses have been more interested in models that are narrowly focused and highly customized for specific tasks. A business application doesn’t need the broad flexibility of something like a consumer-facing chatbot.
“There’s a lot of buzz around general-purpose AI models,” said Grace Yee. “But what’s more important is thinking about how we’re using that technology and whether the use case is high-risk.”
In other words, businesses should think beyond the technology itself and focus on who will use it and how. “Who’s the audience? What’s the intended use case? What’s the domain it’s being used in?” Yee asked.
While larger data sets have traditionally helped improve model performance, there’s growing debate over whether that will always be the case. Some experts suggest that, for certain tasks or populations, adding more data could actually plateau or even hurt performance.
“The idea that bigger data sets automatically lead to better models may be based on flawed assumptions,” said Fernando Diaz and Michael Madaio in their paper, “Scaling Laws Do Not Scale.”
“Models might not keep improving just by feeding them more data — at least not for every group of people or community affected by those models.”
6. AI literacy becomes essential
Generative AI is everywhere now, so understanding how it works has become an important skill for everyone, from top executives to everyday employees. It’s not just about knowing how to use these tools, but also how to evaluate what they produce and, most importantly, how to handle their limitations.
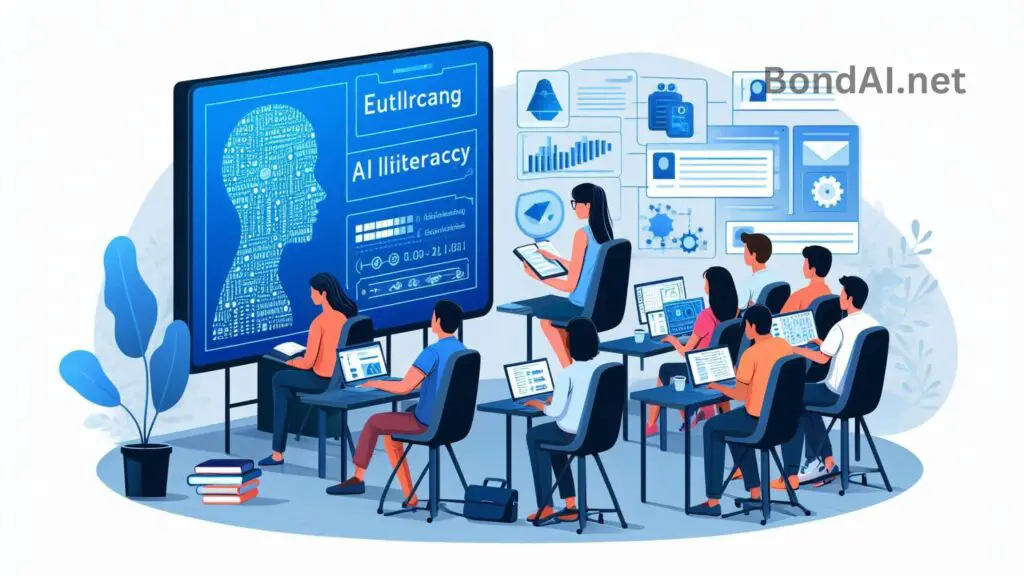
Even though there’s still high demand for AI and machine learning experts, you don’t need to be an AI engineer to get the hang of these tools. “You don’t have to code or train models to understand how to use them,” said Eric Sydell. “Just experimenting, exploring, and using the tools can be incredibly helpful.”
Despite all the buzz around generative AI, it’s still a pretty new technology. Many people haven’t used it at all, or don’t use it regularly. A recent study found that, as of August 2024, less than half of Americans aged 18 to 64 use generative AI, and only a little over a quarter use it for work.
That’s a faster adoption rate than the internet or PCs had, but it still doesn’t reach the majority. Plus, there’s a gap between what businesses say about using generative AI and how it’s actually being used day-to-day by workers.
David Deming, a professor at Harvard University and one of the study’s authors, pointed out that even though many companies claim they’re using AI, only a small percentage are formally integrating it into their operations. Most people are using it informally, like helping write emails, finding information, or looking up how-to guides.
Grace Yee sees a role for both companies and schools in closing the AI skills gap. “Companies get that employees need on-the-job training, because that’s where the work gets done,” she said.
Universities, on the other hand, are starting to focus more on skill-based education, offering learning opportunities that are ongoing and useful across different roles.
“The business world is changing so fast,” said Yee. “You can’t just take time off for a master’s degree anymore. We need to make learning more modular and available to people in real time.”
7. Businesses adjust to an evolving regulatory environment
As 2024 went on, companies found themselves navigating a messy and fast-changing regulatory environment. While the EU introduced new compliance rules with the AI Act in 2024, the U.S. remained largely unregulated, and that trend is likely to continue in 2025 under the Trump administration.
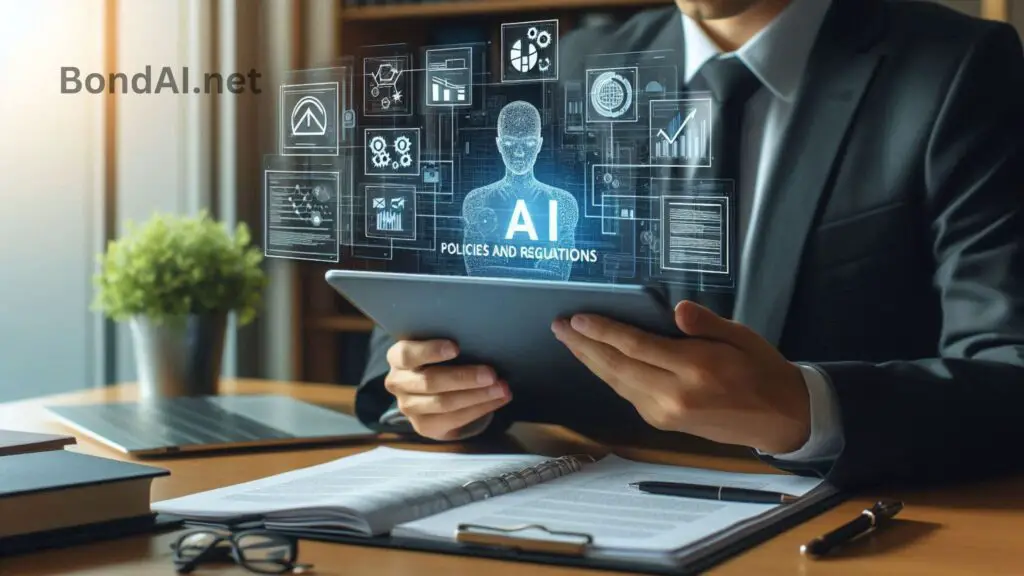
“Right now, the legislation and regulation around these tools is pretty inadequate,” said Eric Sydell. “It doesn’t seem like that’s going to change anytime soon.” Grace Yee agreed, adding that she’s “not expecting much regulation from the new administration.”
This hands-off approach could help drive AI innovation and development, but it also raises concerns about safety and fairness.
Yee believes we need regulations that protect the integrity of online content. This could include giving users access to information about where content comes from, as well as laws to prevent impersonation and protect creators.
To avoid harm without holding back innovation, Yee suggested regulations that match the risk level of specific AI applications. “For low-risk AI, it should be easier to get to market, while high-risk AI would go through a more careful review process,” she explained.
Stave also noted that just because the U.S. has minimal oversight, it doesn’t mean companies can act however they want.
In fact, without a global standard, big companies that operate in multiple regions often follow the strictest regulations by default. So, the EU’s AI Act could end up becoming a global benchmark, much like the GDPR did for data privacy.
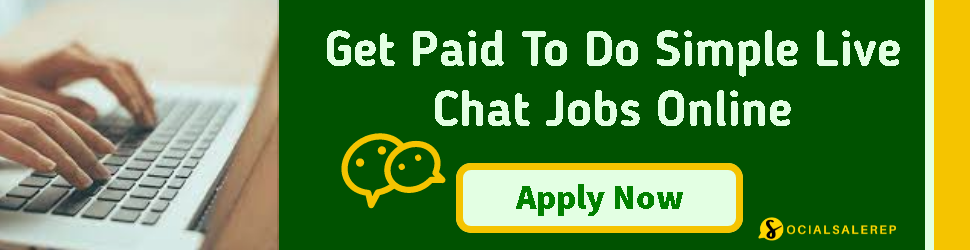
Also Read: What Role Does Blockchain Play in AI Development?
8. AI-related security concerns escalate
Generative AI is now widely available, often for free or at a low cost, giving cybercriminals easy access to powerful tools for launching attacks. This threat is expected to grow in 2025 as multimodal models—AI systems that can handle different types of media—become more advanced and accessible.
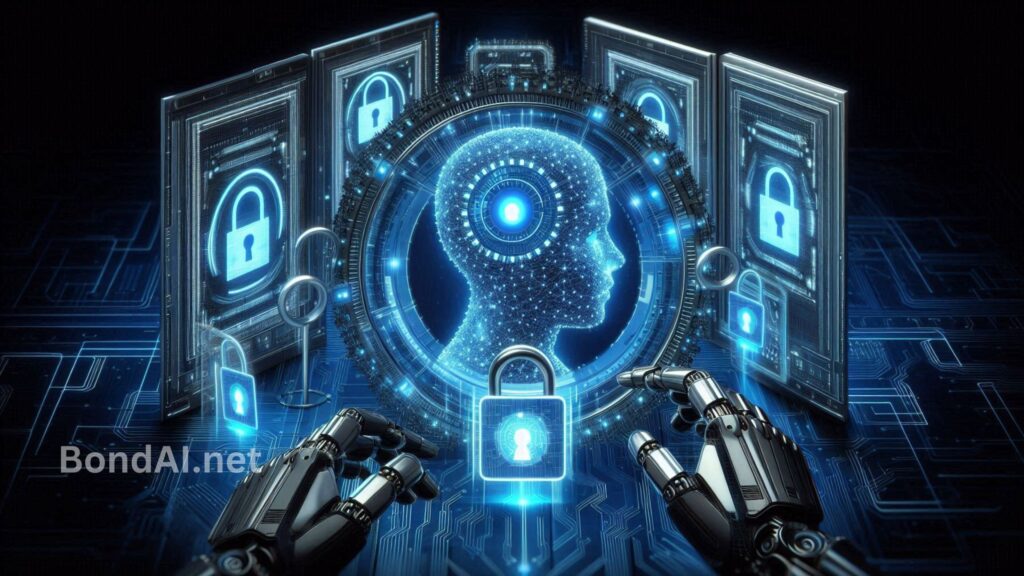
The FBI recently warned that cybercriminals are using generative AI for things like phishing scams and financial fraud. For instance, attackers might create fake social media profiles using AI to write convincing bios and direct messages, or use AI-generated images to make their fake identities seem more real.
AI-generated video and audio are becoming an even bigger problem. In the past, these models were easy to spot because they sounded robotic or had glitchy video, but today’s versions are much better. If a victim is in a rush or not paying close attention, these AI-generated messages can be hard to spot.
Audio tools let hackers impersonate a victim’s trusted contacts, like a spouse or coworker. Video deepfakes are less common, mostly because they’re more expensive to create and harder to perfect.
However, in a highly publicized case earlier this year, scammers successfully used deepfakes to impersonate a company’s CFO and other staff on a video call, tricking a finance worker into sending $25 million to fraudulent accounts.
There are also security risks tied directly to vulnerabilities in the AI models themselves. Hackers can use techniques like adversarial machine learning or data poisoning to mess with AI systems by feeding them misleading or corrupt data. To tackle these threats, businesses will need to make AI security a key part of their overall cybersecurity plans.